- Ilya Sutskever has been a strong advocate for scaling LLMs as a path to unlocking greater intelligence.
- Now, Sutskever says that LLM scaling has plateaued. It's now time to scale the right thing.
- Similar to OpenAI's o1 models, Google and Anthropic are working on inference scaling techniques.
While OpenAI chief Sam Altman is drumming up hype that AGI is just around the corner, new reports suggest that LLM scaling has hit a wall. The predominant view in the AI field has been that training larger models on massive amounts of data and compute resources will lead to greater intelligence.
In fact, Ilya Sutskever, former chief scientist at OpenAI and founder of Safe Superintelligence Inc., has been a strong advocate for scaling models as the path to unlocking intelligence. Responding to Reuters, Sutskever now says, “results from scaling up pre-training – the phase of training an AI model that uses a vast amount of unlabeled data to understand language patterns and structures – have plateaued.”
In a turnaround, Sutskever says scaling the right things now matters: “The 2010s were the age of scaling, now we’re back in the age of wonder and discovery once again. Everyone is looking for the next thing. Scaling the right thing matters more now than ever.”
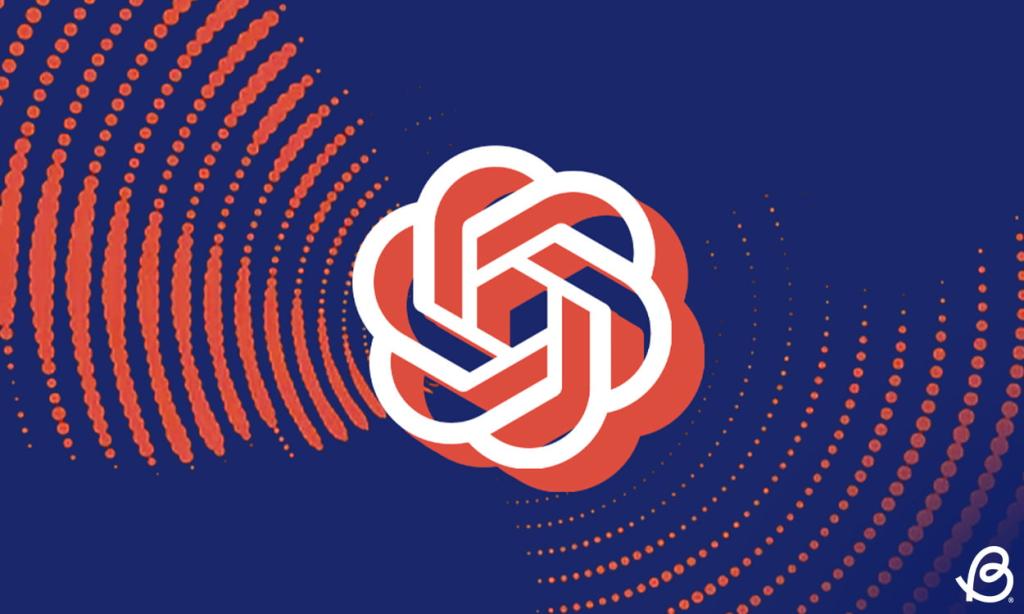
That’s the reason OpenAI released its new series of ’01’ reasoning models on ChatGPT that scale during inference. It has been seen that if AI models are given more time to “think” and re-evaluate their response, they yield far better results. So companies are now focusing more on test-time compute which means adding more resources during inference and then generating a final response.
Recently, The Information reported that OpenAI has changed its strategy as its next big “Orion” model didn’t deliver better results as anticipated. The jump from GPT-3.5 to GPT-4 was huge, but OpenAI employees who tested the upcoming model say that the improvement from GPT-4 to Orion is marginal. In tasks like coding, it doesn’t outperform prior GPT models.
OpenAI is now focused on inference scaling as a new way to improve model performance on ChatGPT. Noam Brown, a researcher at OpenAI, says that inference scaling improves the model performance significantly.
Recently, he tweeted, “OpenAI’s o1 thinks for seconds, but we aim for future versions to think for hours, days, even weeks. Inference costs will be higher, but what cost would you pay for a new cancer drug? For breakthrough batteries? For a proof of the Riemann Hypothesis? AI can be more than chatbots.”
Google and Anthropic are also working on a similar technique to improve model performance through inference scaling. However, François Chollet, a researcher at Google, argues that scaling LLMs alone won’t lead to generalized intelligence. Yann LeCun, chief AI scientist at Meta, similarly says that LLMs are not sufficient for achieving AGI.
As companies run out of data to train larger models, they are looking for novel techniques to improve LLM performance. Now whether AGI is genuinely around the corner or it’s simply hype is something only time will tell.